AFFILIATE RESEARCH
Multimodal Drivers of Attention Interruption to Baby Product Video Ads
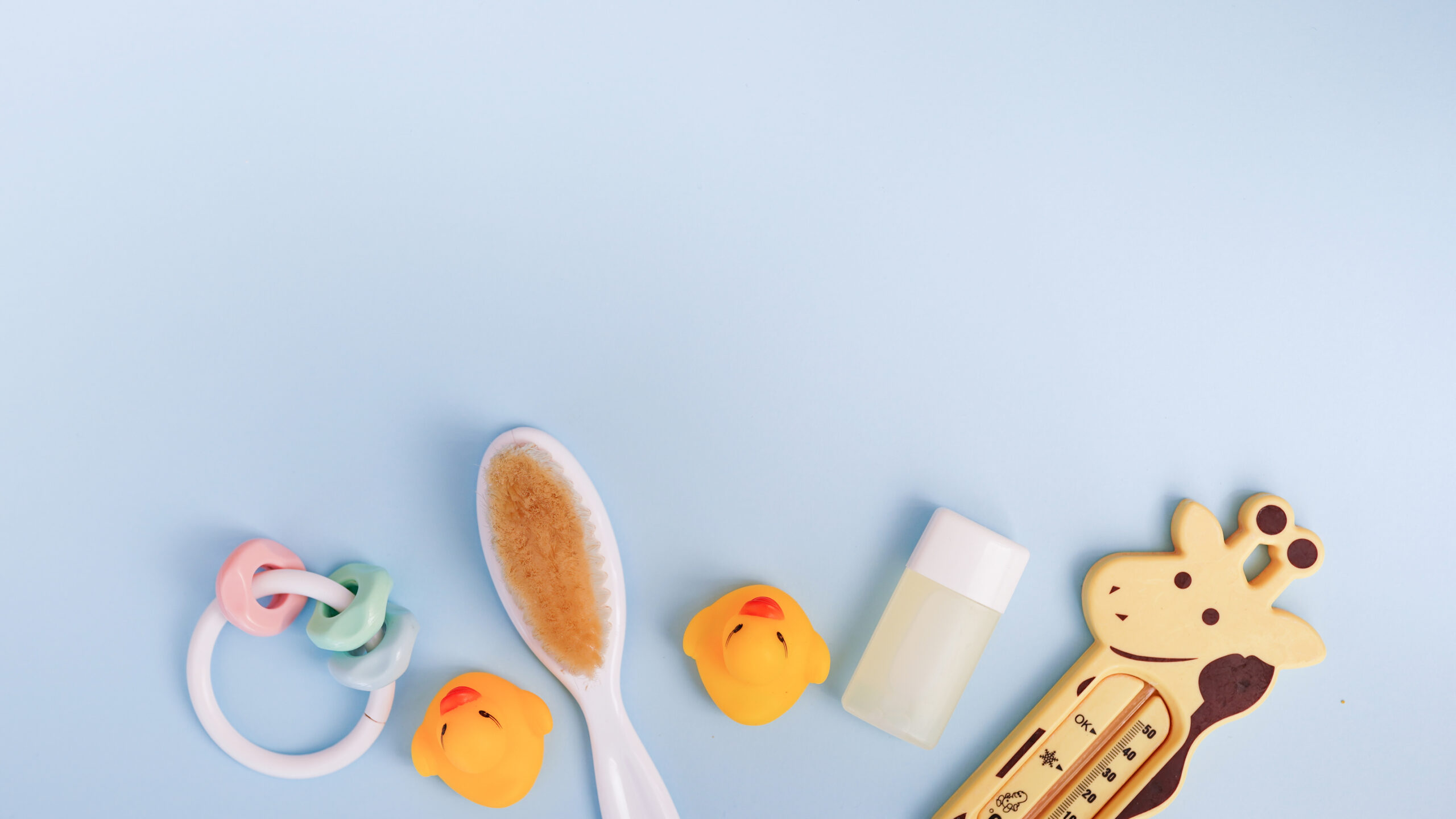
By Yakov Bart | December 2024
Ad designers often use sequences of shots in video ads, where frames are similar within a shot but vary across shots. These visual variations, along with changes in auditory and narrative cues, can interrupt viewers’ attention. In this paper, we address the underexplored task of applying multimodal feature extraction techniques to marketing problems. We introduce the “AttInfaForAd” dataset, containing 111 baby product video ads with visual ground truth labels indicating points of interest in the first, middle, and last frames of each shot, identified by 75 shoppers. We propose attention interruption measures and use multimodal techniques to extract visual, auditory, and linguistic features from video ads. Our feature-infused model achieved the lowest mean absolute error and highest R-square among various machine learning algorithms in predicting shopper attention interruption. We highlight the significance of these features in driving attention interruption. By open-sourcing the dataset and model code, we aim to encourage further research in this crucial area. Learn More >>
Other Affiliate Research

A Case Study in an A.I.-Assisted Content Audit
This paper presents an experimental case study utilizing machine learning and generative AI to audit content diversity in a hyper- local news outlet, The Scope, based at a university and focused on underrepresented communities in Boston. Through computational text analysis, including entity extraction, topic labeling, and quote extraction and attribution, we evaluate the extent to which The Scope’s coverage aligns with its mission to amplify diverse voices.
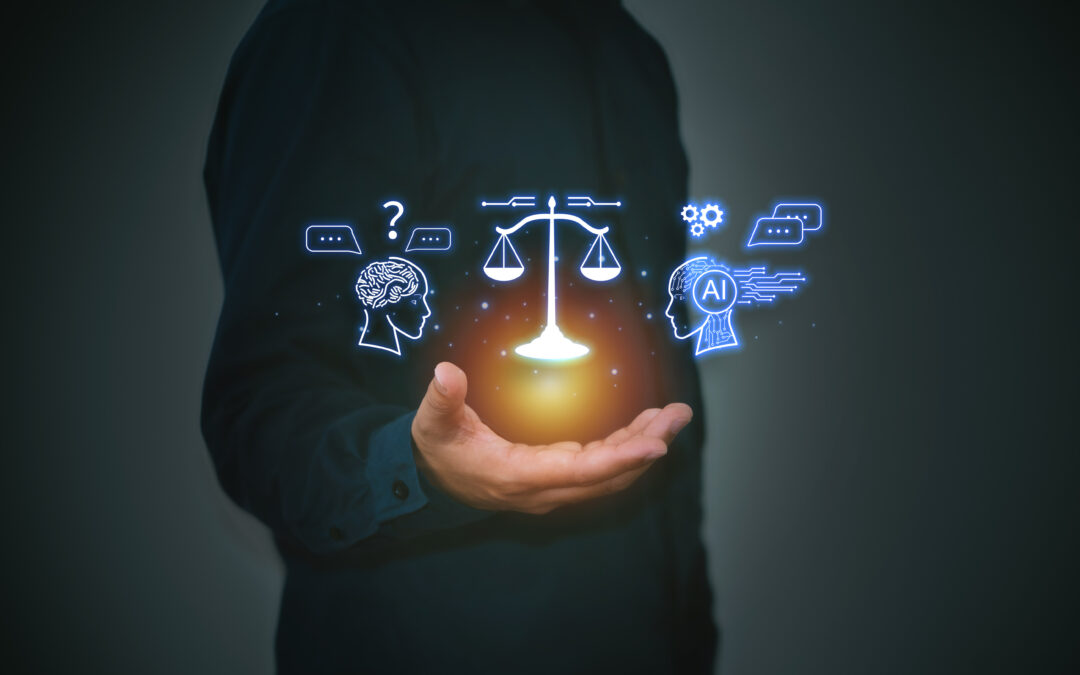
AI Regulation: Competition, Arbitrage & Regulatory Capture
The commercial launch of ChatGPT in November 2022 and the fast development of Large Language Models catapulted the regulation of Artificial Intelligence to the forefront of policy debates One overlooked area is the political economy of these regulatory initiatives–or how countries and companies can behave strategically and use different regulatory levers to protect their interests in the international competition on how to regulate AI.
This Article helps fill this gap by shedding light on the tradeoffs involved in the design of AI regulatory regimes in a world where: (i) governments compete with other governments to use AI regulation, privacy, and intellectual property regimes to promote their national interests; and (ii) companies behave strategically in this competition, sometimes trying to capture the regulatory framework.
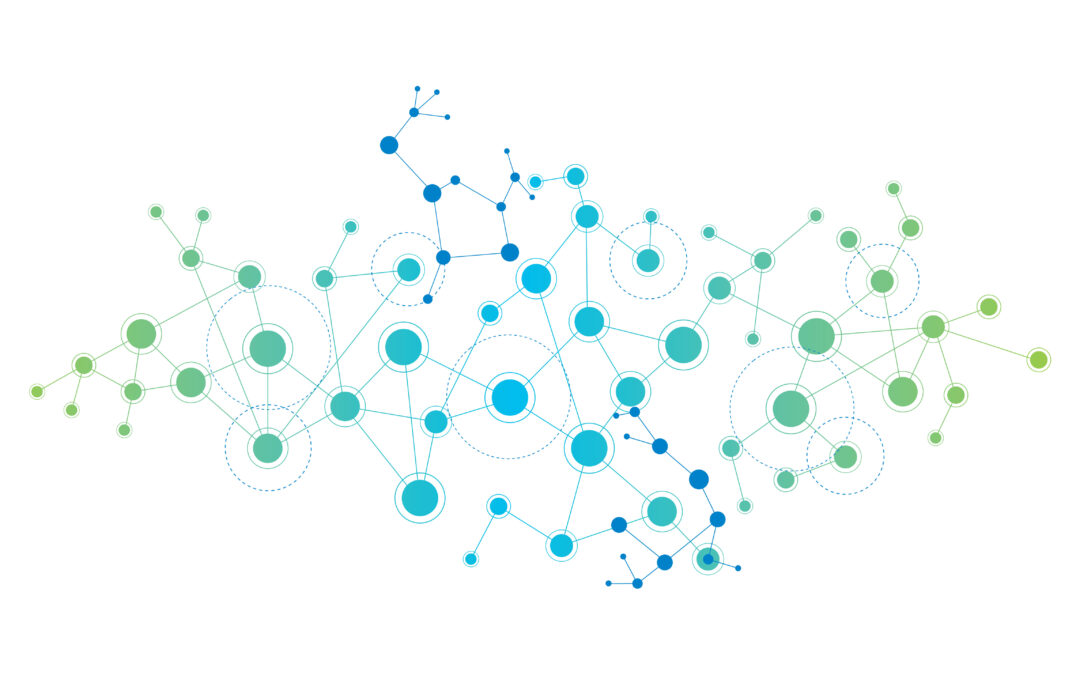
When Randomness Beats Redundancy: Insights into the Diffusion of Complex Contagions
How does social network structure amplify or stifle behavior diffusion? Existing theory suggests that when social reinforcement makes the adoption of behavior more likely, it should spread more — both farther and faster — on clustered networks with redundant ties. Conversely, if adoption does not benefit from social reinforcement, then it should spread more on random networks without such redundancies. We develop a novel model of behavior diffusion with tunable probabilistic adoption and social reinforcement parameters to systematically evaluate the conditions under which clustered networks better spread a behavior compared to random networks.
Tags:
Contact Us
Are you interested in joining the IDI team or have a story to tell? reach out to us at j.wihbey@northeastern.edu