THE INTERNET DEMOCRACY INITIATIVE
Recent Publications
Internet Democracy Initiative affiliates conduct research at the intersection of technology, media, and politics.
Search Articles
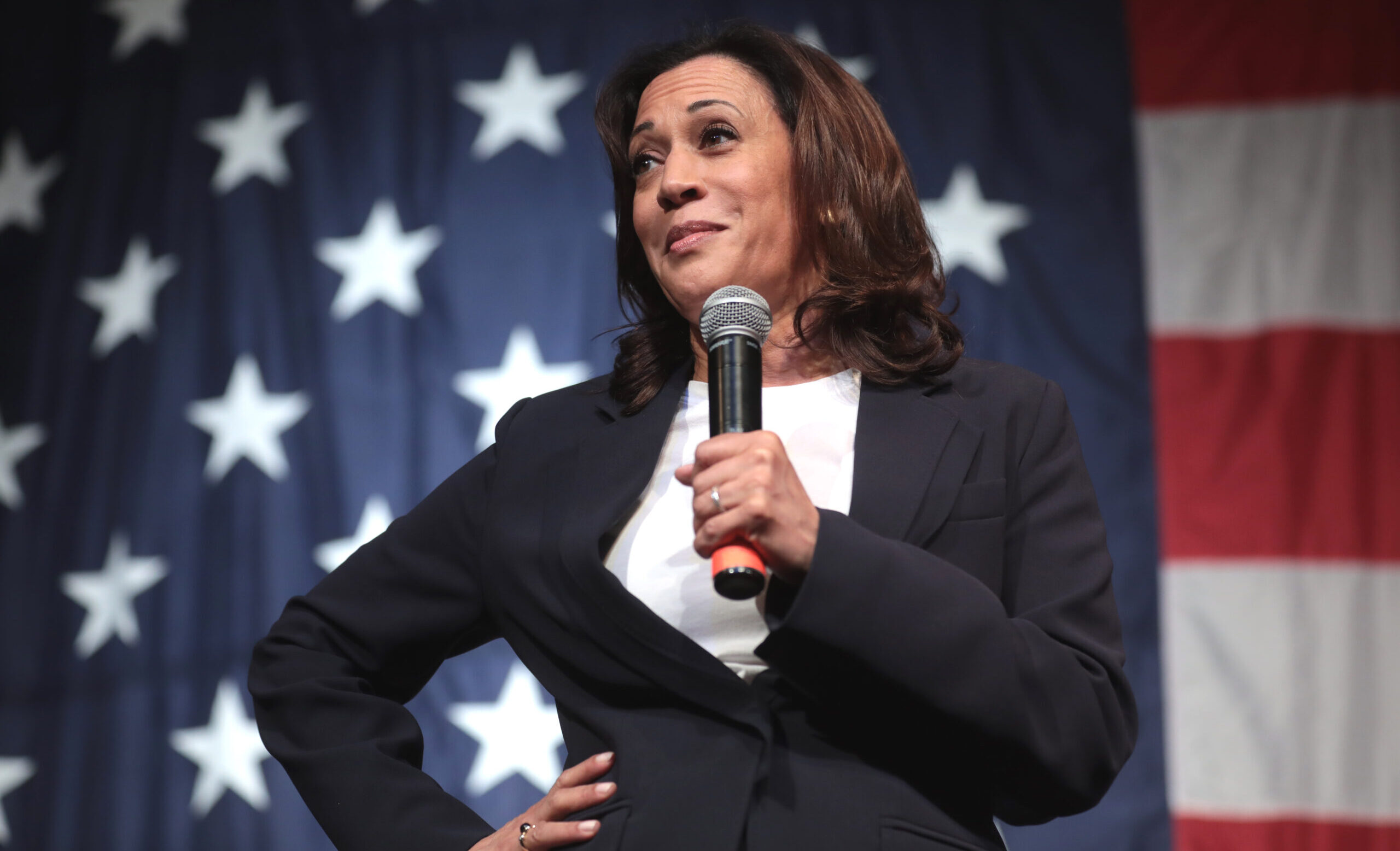
November 2023
Powerful in Pearls and Willie Brown’s Mistress: a Computational Analysis of Gendered News Coverage of Kamala Harris on the Partisan Extremes
Meg Heckman, Rahul Bhargavaa, and Emily Boardman Ndulue
This study uses a mix of traditional and computational content analysis to track digital news coverage of U.S. Vice President Kamala Harris during and immediately after the 2020 general elections. It’s well documented that female politicians, especially women of color, face biased coverage in the political press. In this paper, we explore how these dynamics play out in the modern hyper-polarized digital media landscape. Our corpus includes roughly 17,000 stories published online between August 2020 and April 2021 by news organizations we categorized along a binary axis of partisanship between Republican and Democrat. Our findings show that sexist, racialized coverage of Harris is most prevalent in news sources shared by mostly Republican voters. Coverage in news sources shared by registered Democratic voters, meanwhile, tended to treat Harris as a celebrity, often fixating on her wardrobe and personal life. We ponder implications for both gender equity in civic life and future feminist media scholarship.
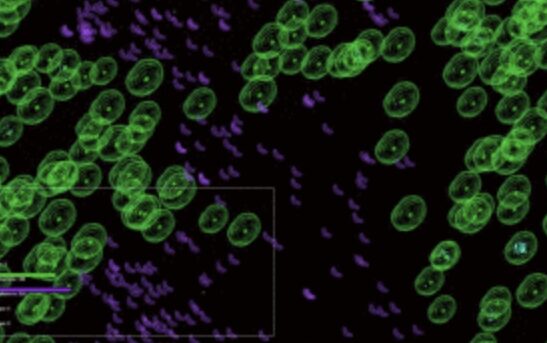
September 2023
Interactive Visualization of Plankton – Mediated Nutrient Cycling in the Narragansett Bay
Avantika Velho, Pedro Cruz, David Banks-Richardson, Gabrielle Armin, Ying Zhang, Keisuke Inomura, and Katia Zolotovsky
Microbial plankton play a critical role in global biogeochemical cycling, and while a vast amount of genomics data has been collected from microbial communities throughout the ocean, there remains a gap in connecting this data to ecosystems and biogeochemical cycles. One key question is how different genotypes influence an organism’s environmental impact? Here, we scientists and designers have collaborated to develop an interactive visual tool that helps to better understand the relationships between microbial ecosystems and global biogeochemical cycling.
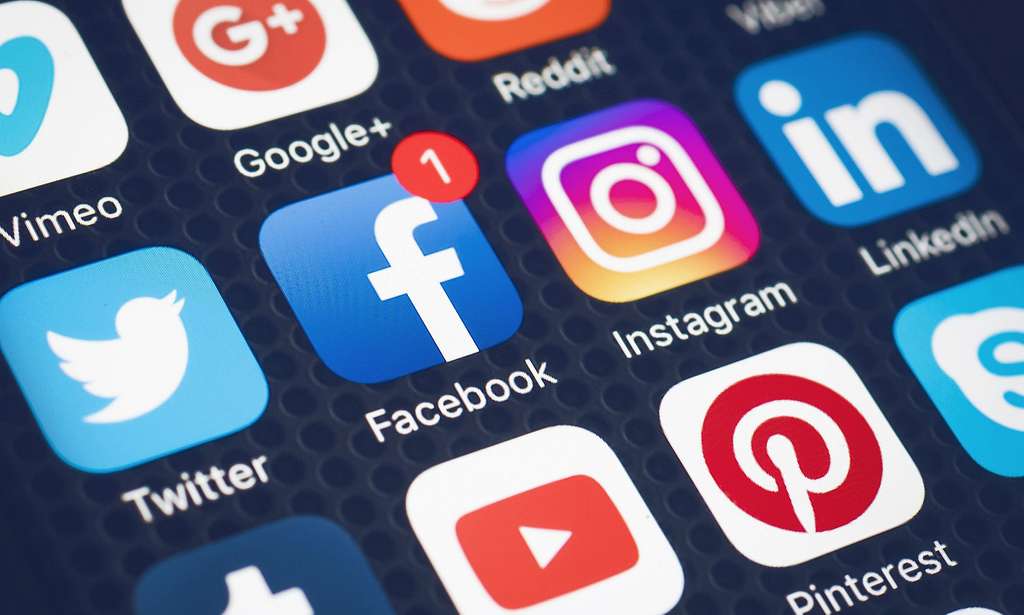
August 2023
Measuring Disparate Outcomes of Content Recommendation Algorithms with Distributional Inequality Metrics
c
The harmful impacts of algorithmic decision systems have recently come into focus, with many examples of machine learning (ML) models amplifying societal biases. In this paper, we propose adapting income inequality metrics from economics to complement existing model-level fairness metrics, which focus on intergroup differences of model performance. In particular, we evaluate their ability to measure disparities between exposures that individuals receive in a production recommendation system, the Twitter algorithmic timeline. We define desirable criteria for metrics to be used in an operational setting by ML practitioners. We characterize engagements with content on Twitter using these metrics and use the results to evaluate the metrics with respect to our criteria. We also show that we can use these metrics to identify content suggestion algorithms that contribute more strongly to skewed outcomes between users. Overall, we conclude that these metrics can be a useful tool for auditing algorithms in production settings.
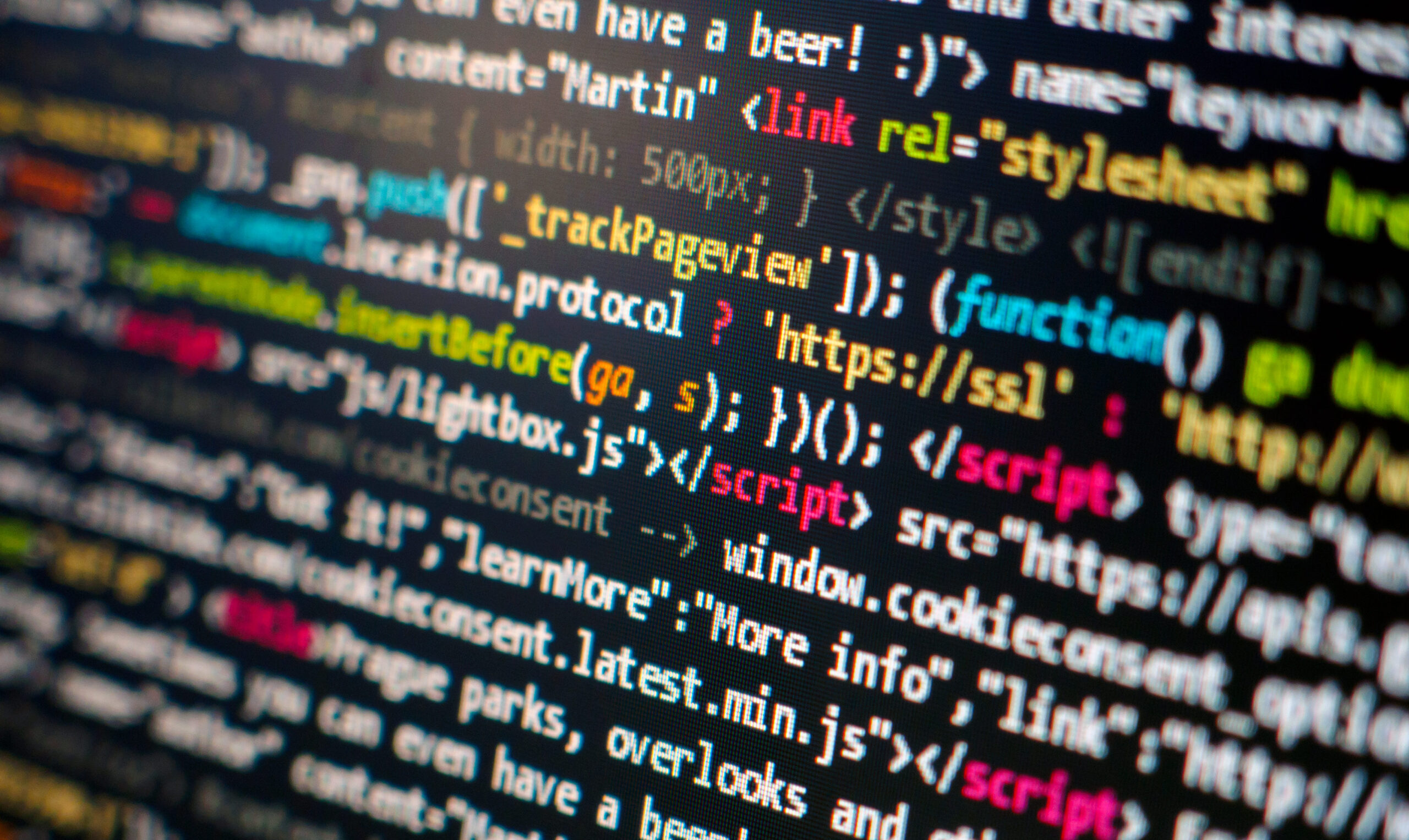
August 2023
What’s in the Black Box? How Algorithmic Knowledge Promotes Corrective and Restrictive Actions to Counter Misinformation in the USA, the UK, South Korea and Mexico
While there has been a growing call for insights on algorithms given their impact on what people encounter on social media, it remains unknown how enhanced algorithmic knowledge serves as a countermeasure to problematic information flow. To fill this gap, this study aims to investigate how algorithmic knowledge predicts people’s attitudes and behaviors regarding misinformation through the lens of the third-person effect.
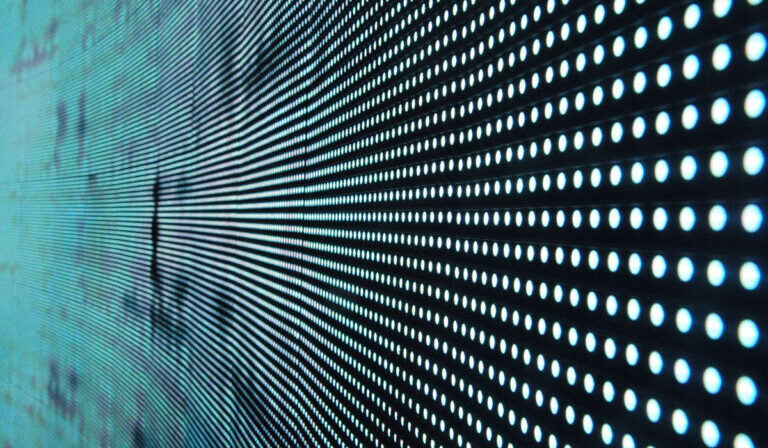
August 2023
What We Owe to Decision-Subjects: Beyond Transparency and Explanation in Automated Decision-making
David Gray Grant, Jeff Behrends, and John Basl
The ongoing explosion of interest in artificial intelligence is fueled in part by recently developed techniques in machine learning. Those techniques allow automated systems to process huge amounts of data, utilizing mathematical methods that depart from traditional statistical approaches, and resulting in impressive advancements in our ability to make predictions and uncover correlations across a host of interesting domains. But as is now widely discussed, the way that those systems arrive at their outputs is often opaque, even to the experts who design and deploy them. Is it morally problematic to make use of opaque automated methods when making high-stakes decisions, like whether to issue a loan to an applicant, or whether to approve a parole request? Many scholars answer in the affirmative. However, there is no widely accepted explanation for why transparent systems are morally preferable to opaque systems. We argue that the use of automated decision-making systems sometimes violates duties of consideration that are owed by decision-makers to decision-subjects, duties that are both epistemic and practical in character. Violations of that kind generate a weighty consideration against the use of opaque decision systems. In the course of defending our approach, we show that it is able to address three major challenges sometimes leveled against attempts to defend the moral import of transparency in automated decision-making.
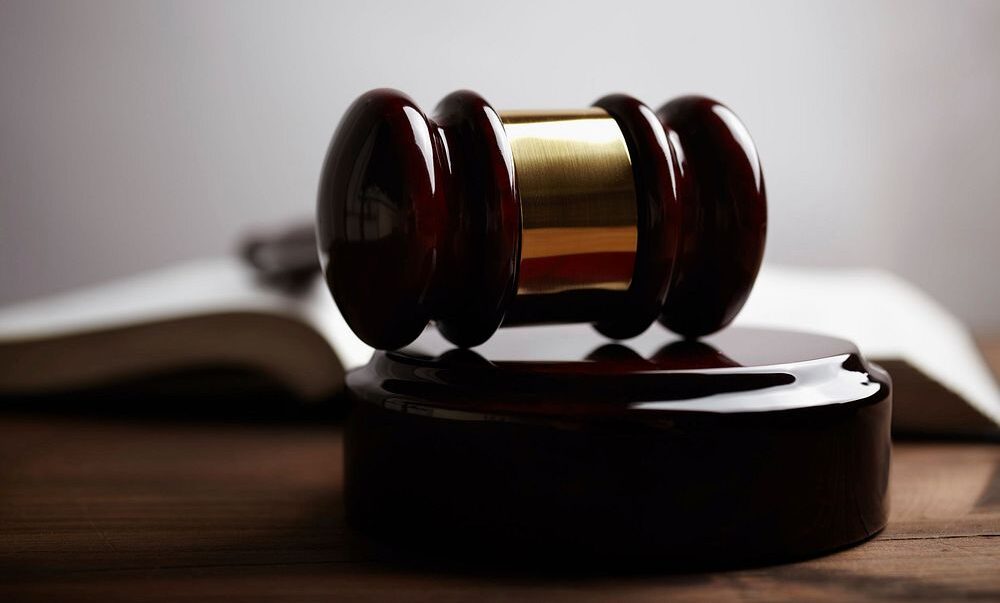
August 2023
Rawls and Antitrust’s Justice Function
Antitrust law is more contested than ever. The recent push by the Biden Administration to re-orient antitrust towards justice and fairness considerations is leading to public backlash, judicial resistance and piecemeal doctrinal developments. The methodological hegemony of welfare maximizing moves in antitrust makes it theoretically fragile and maladaptive to change. To bridge disagreements and overcome polarization, this Article revisits John Rawls’ foundational work on political and economic justice, arguing that it can facilitate consensus and inform the present and future of antitrust law.
John Rawls’ work on justice can help re-orient antitrust law in four ways. First, it provides a common language of justice that enables various “camps” of thinkers and activists find common ground on the relation between antitrust’s economic mission and its implications for political justice. Second, it helps recalibrate the balance between utilitarian moves that emphasize welfare maximization on the one hand and deontological or structural justice considerations on the other. Third, and related, Rawls guides our thinking on the relationship between justice, efficiency and welfare, showing us that attempts at separating antitrust law’s mission from its impact on liberty, equality and fairness violates basic moral intuitions. Fourth, Rawls’ late work on political economy, particularly notions of property-owning democracy and liberal democratic socialism, can help situate antitrust law within broader policy thinking on economic justice.
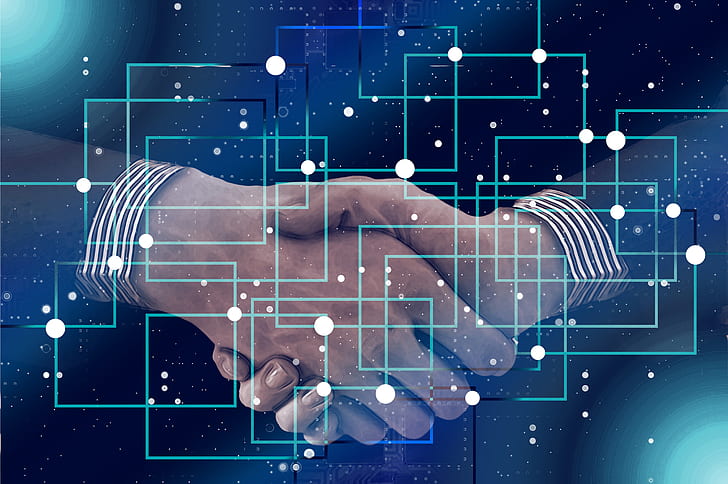
July 2023
Enhancing the Ethics of User-Sourced Online Data Collection and Sharing
Michelle N. Meyer, John Basl, David Choffnes, Christo Wilson, and David M. J. Lazer
Social media and other internet platforms are making it even harder for researchers to investigate their effects on society. One way forward is user-sourced data collection of data to be shared among many researchers, using robust ethics tools to protect the interests of research participants and society.
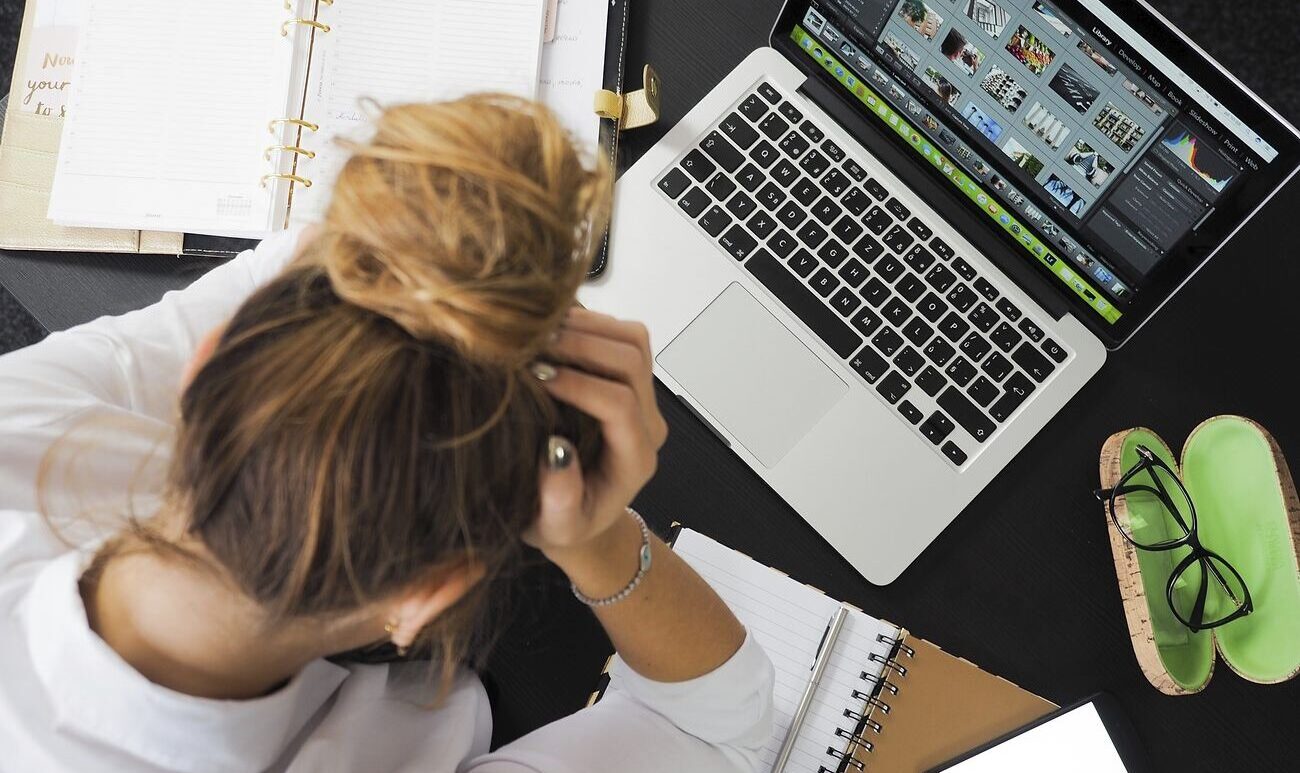
June 2023
An Example of (Too Much) Hyper-Parameter Tuning In Suicide Ideation Detection
Annika Marie Schoene, John Ortega, Silvio Amir, and Kenneth Church
This work starts with the TWISCO baseline, a benchmark of suicide-related content from Twitter. We find that hyper-parameter tuning can improve this baseline by 9%. We examined 576 combinations of hyper-parameters: learning rate, batch size, epochs and date range of training data. Reasonable settings of learning rate and batch size produce better results than poor settings. Date range is less conclusive. Balancing the date range of the training data to match the benchmark ought to improve performance, but the differences are relatively small. Optimal settings of learning rate and batch size are much better than poor settings, but optimal settings of date range are not that different from poor settings of date range. Finally, we end with concerns about reproducibility. Of the 576 experiments, 10% produced F1 performance above baseline. It is common practice in the literature to run many experiments and report the best, but doing so may be risky, especially given the sensitive nature of Suicide Ideation Detection.
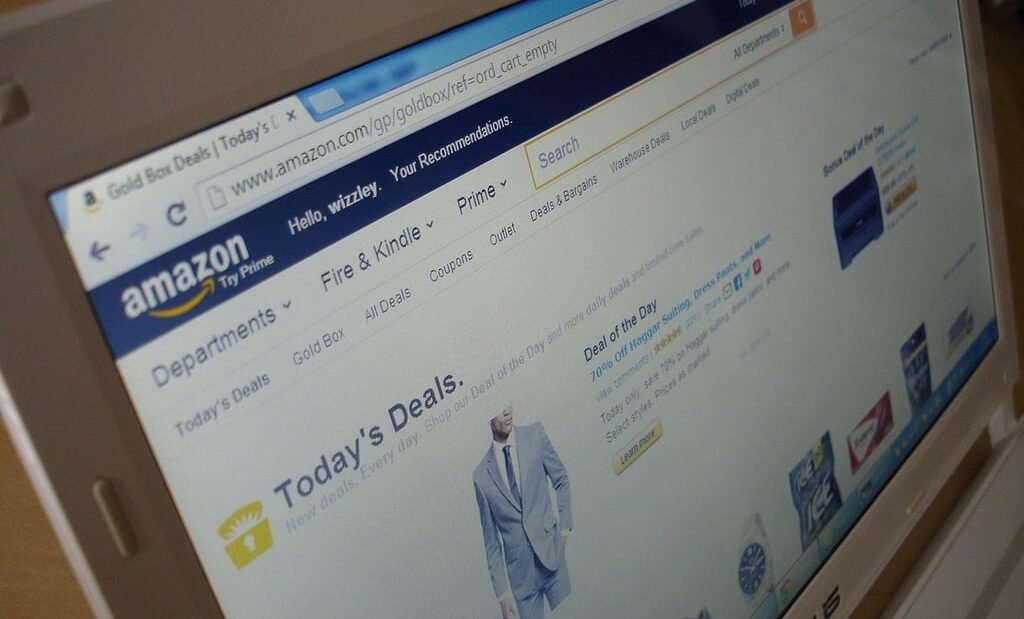
December 2023
Generative AI and User-Generated Content: Evidence from Online Reviews
Samsun Knight and Yakov Bart
How has generative artificial intelligence affected the quality and quantity of user-generated content? Our analysis of restaurant reviews on Yelp.com and product reviews on Amazon.com shows that, contrary to prior lab evidence, use of generative AI in text generation is associated with significant declines in online content quality. These results are similar both in OLS and in two- period differences-in-differences estimation based on within-reviewer changes in AI use. We also find that use of generative AI is associated with increases in per-reviewer quantity of content, and document heterogeneity in the observed effect between expert and non-expert reviewers, with the strongest declines in quality associated with use by non-experts.